BUILDING FRONTIER AI
BUILDING FRONTIER AI
BUILDING FRONTIER AI
FOR DOCUMENT
FOR DOCUMENT
FOR DOCUMENT
AND DATA PROCESSING
AND DATA PROCESSING
AND DATA PROCESSING
IN REGULATED INDUSTRIES
IN REGULATED INDUSTRIES
IN REGULATED INDUSTRIES
OUR MODELS
OUR MODELS
COMPLIANT WITH THE EU LEGISLATION
COMPLIANT WITH THE EU LEGISLATION
COMPLIANT WITH THE EU LEGISLATION
ENERGETICALLY EFFICIENT
ENERGETICALLY EFFICIENT
ENERGETICALLY EFFICIENT
EXCELLING AT MULTILINGUAL USE
EXCELLING AT MULTILINGUAL USE
EXCELLING AT MULTILINGUAL USE
AI ASSISTANTS FOR REGULATED INDUSTRIES AND SENSITIVE USE CASES
AI ASSISTANTS FOR REGULATED INDUSTRIES AND SENSITIVE USE CASES
(01)
DESIGNED FOR COMPLEX DOCUMENT PROCESSING, RAG AND DATA HARMONISATION
Excelling at enterprise, knowledge intensive tasks requiring analysis and transformation of multimodal long context (32k+) documents and data fast and with highest degree of accuracy.
(01)
DESIGNED FOR COMPLEX DOCUMENT PROCESSING, RAG AND DATA HARMONISATION
Excelling at enterprise, knowledge intensive tasks requiring analysis and transformation of multimodal long context (32k+) documents and data fast and with highest degree of accuracy.
(02)
OPTIMISED FOR LOCAL, FULLY SECURE ENTERPRISE USE
Built for inference on consumer GPUs and CPUs, our assistants can be deployed locally as well as on private clouds, with the possibility of local storage of your documents embeddings. The assistants can be used in fully offline mode for the vast majority of tasks, with only external LLM-powered data collection (scrapping) functionalities requiring to the online mode.
(02)
OPTIMISED FOR LOCAL, FULLY SECURE ENTERPRISE USE
Built for inference on consumer GPUs and CPUs, our assistants can be deployed locally as well as on private clouds, with the possibility of local storage of your documents embeddings. The assistants can be used in fully offline mode for the vast majority of tasks, with only external LLM-powered data collection (scrapping) functionalities requiring to the online mode.
(03)
EXPLAINABLE AND AUDITABLE
Our assistants’ generations cite the documents with exact citations that it bases itself on to allow knowledge workers to verify, to reuse and to go further with the existing enterprise data.
(03)
EXPLAINABLE AND AUDITABLE
Our assistants’ generations cite the documents with exact citations that it bases itself on to allow knowledge workers to verify, to reuse and to go further with the existing enterprise data.
USE CASES
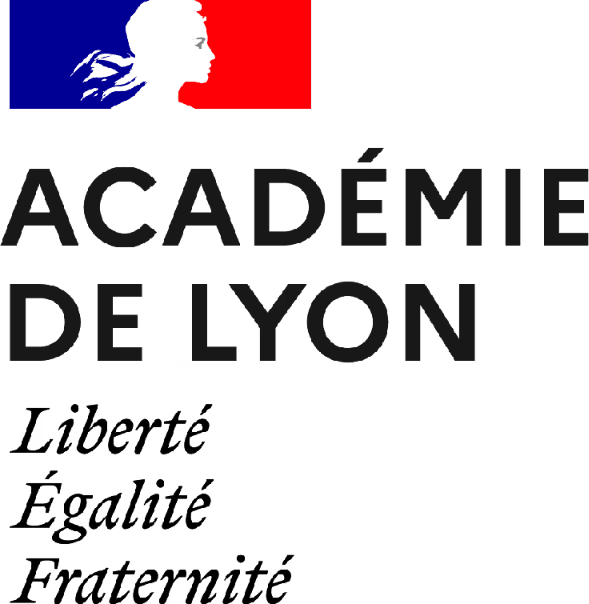
CASSANDRE
A local explainable LLM assistant for HR services
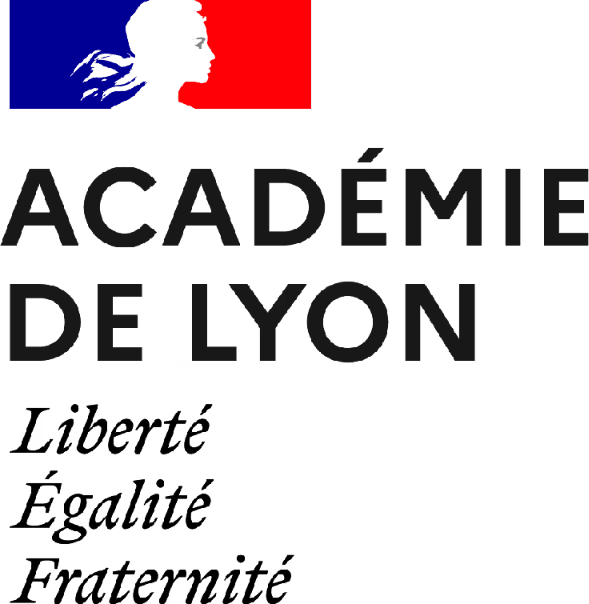
CASSANDRE
A local explainable LLM assistant for HR services
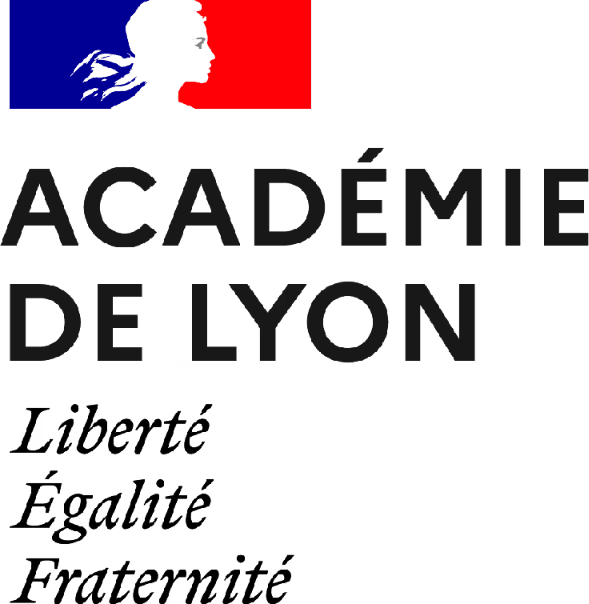
CASSANDRE
A local explainable LLM assistant for HR services
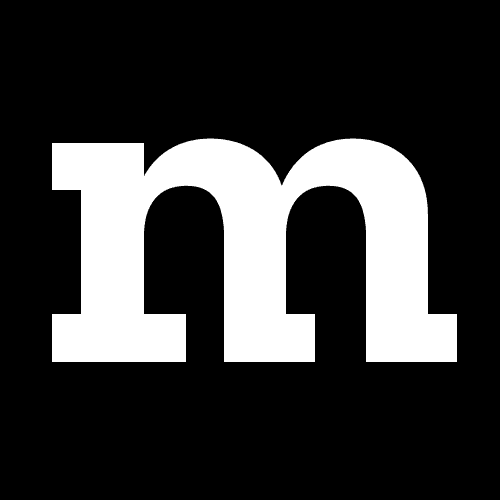
SCHOLASTIC AI
A local R&D assistant with the support of mozilla foundation
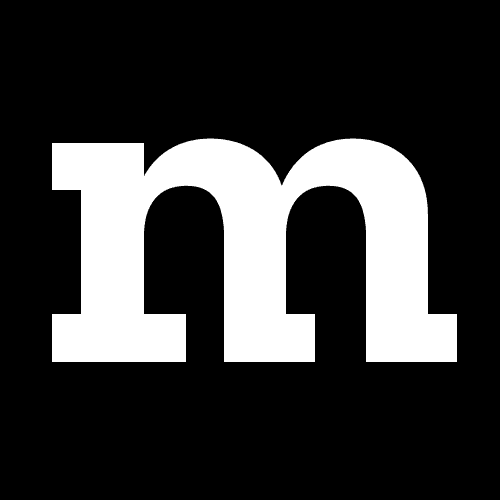
SCHOLASTIC AI
A local R&D assistant with the support of mozilla foundation
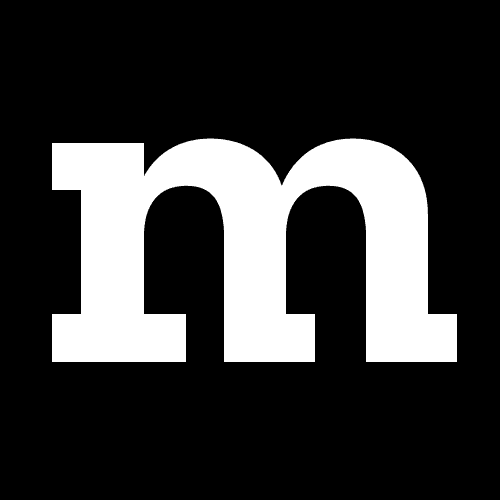
SCHOLASTIC AI
A local R&D assistant with the support of mozilla foundation
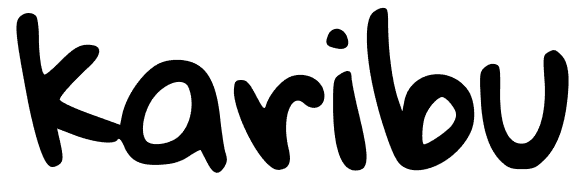
KARIBU
An Educational On-Device Assistant for Senegalese Teachers
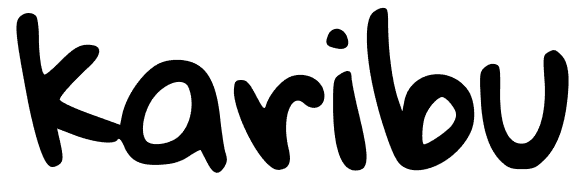
KARIBU
An Educational On-Device Assistant for Senegalese Teachers
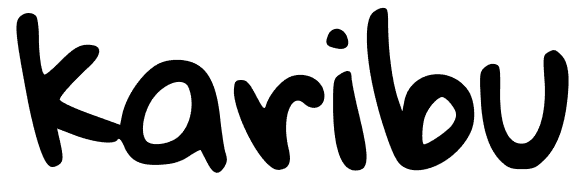
KARIBU
An Educational On-Device Assistant for Senegalese Teachers
FOUNDING TEAM
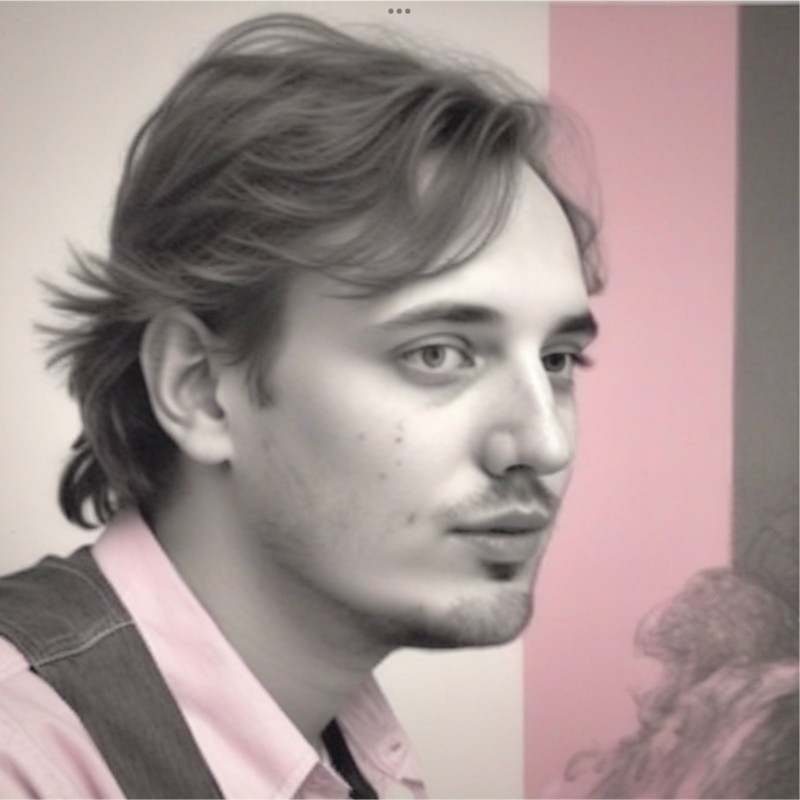
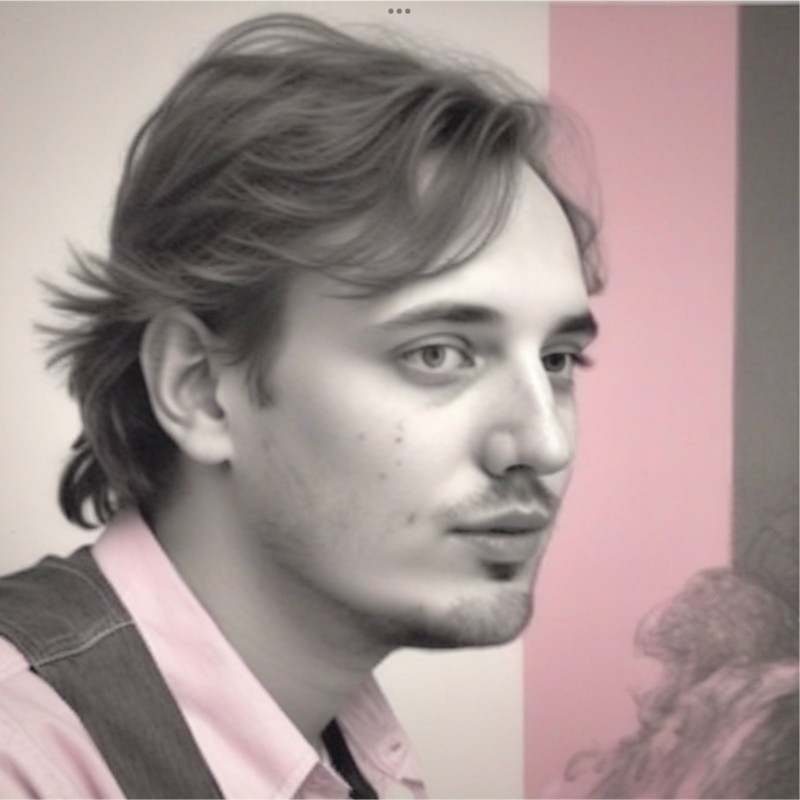
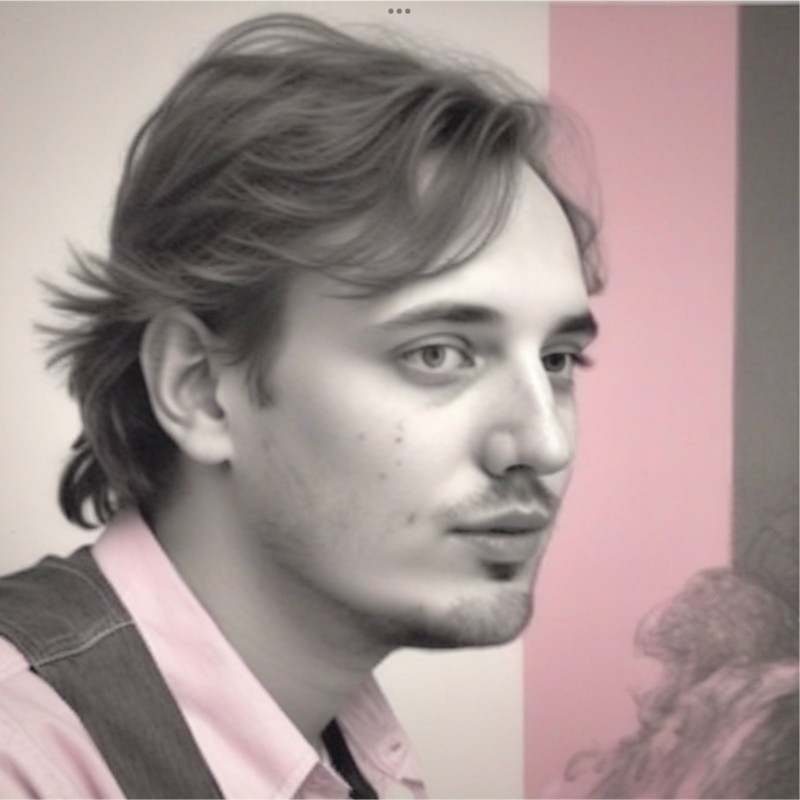
PIERRE-CARL-LANGLAIS
01
PhD Information science
02
Associate Researcher at Sorbonne Center for Artificial Intelligence and Sciences Po Médialab
03
Ex-opsci.ai, Head of Research
pierre-carl@pleias.fr
pierre-carl@pleias.fr
pierre-carl@pleias.fr
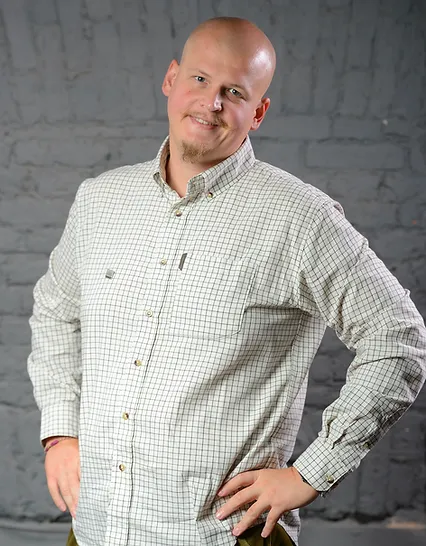
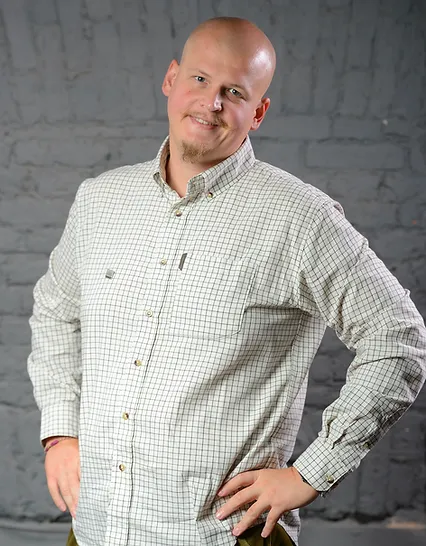
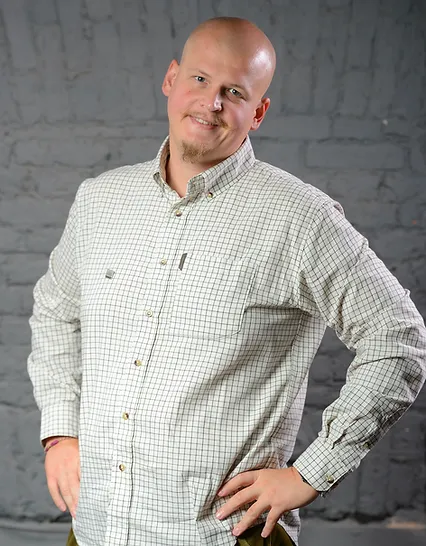
IVAN YAMSCHIKOV
01
PhD Financial Mathematics
02
Associate Researcher at Sorbonne Research Professor at CAIRO, Technical University of Applied Sciences Würzburg for Artificial Intelligence and Sciences Po Médialab
03
Ex-Yandex, R&D Lead in Human-Machine interaction ex-ABBYY, AI Evangelist
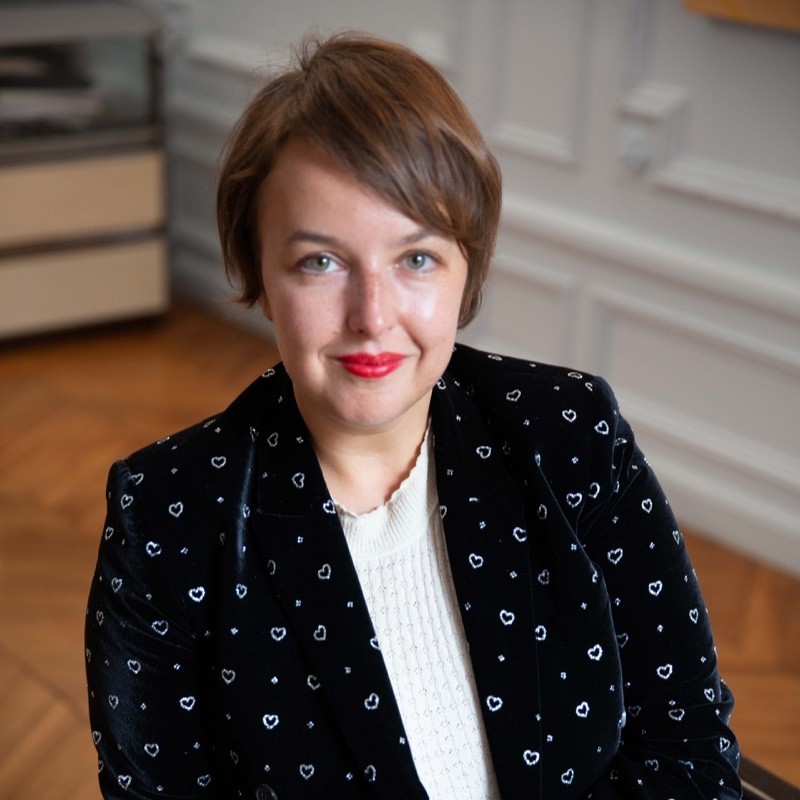
ANASTASIA STASENKO
01
PhD Philosophy, ex-ENS Ulm
02
Associate ResearcherAssociate Senior Lecturer at Sorbonne-Nouvelle Universityat Sorbonne Center for Artificial Intelligence and Sciences Po Médialab
03
Ex-opsci.ai, ex-Hachette Livre, Digital Learning Product Manager of Research
04
Ex-opsci.ai, Research Lead
TEAM
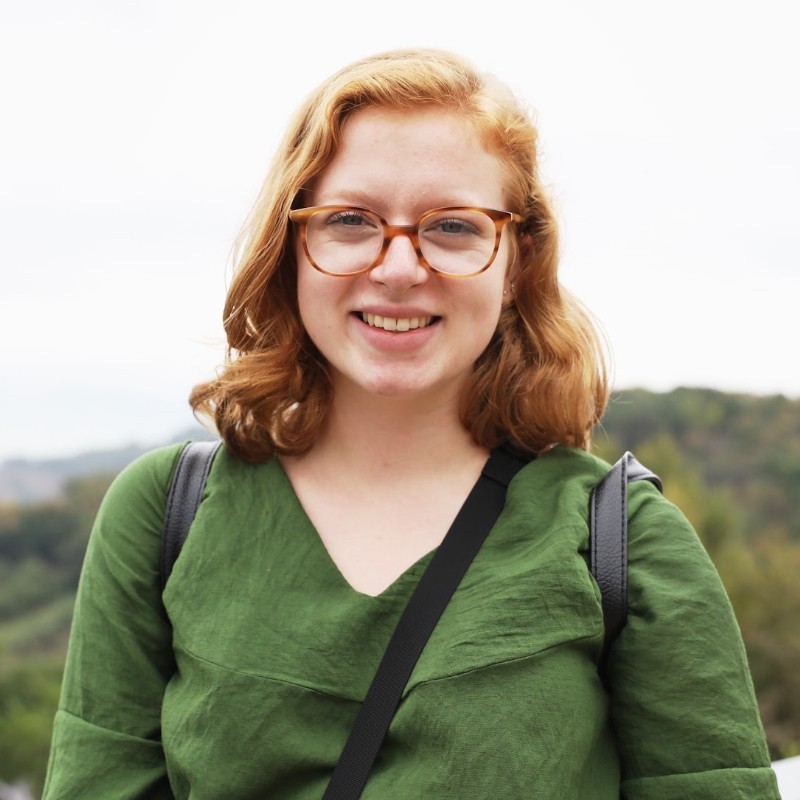
DR CATHERINE ARNETT
LEAD RESEARCH SCIENTIST
Ex-UC San Diego
Multiple papers accepted at EMNLP
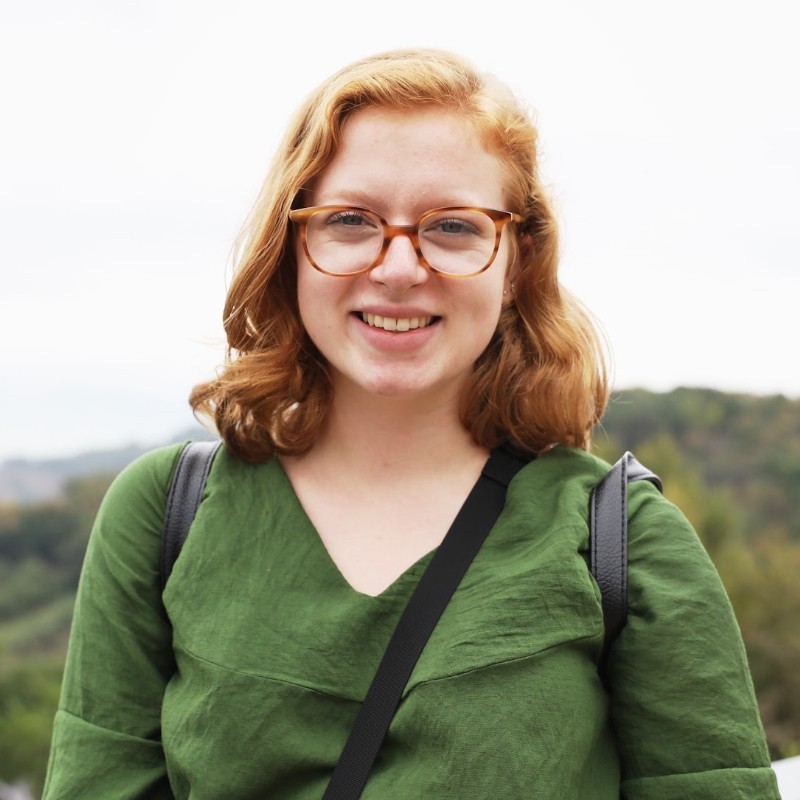
DR CATHERINE ARNETT
LEAD RESEARCH SCIENTIST
Ex-UC San Diego
Multiple papers accepted at EMNLP
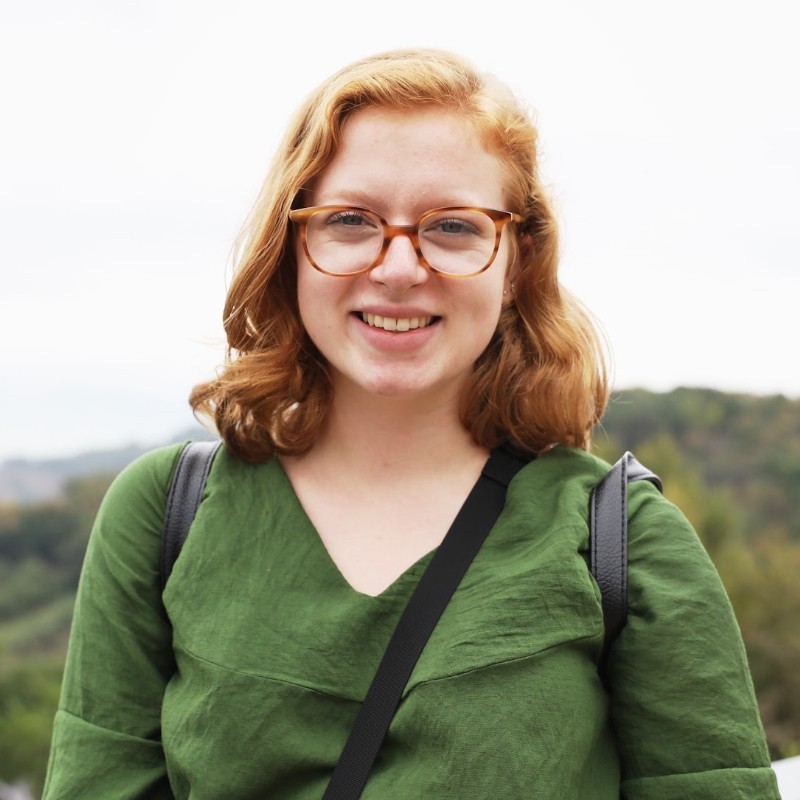
DR CATHERINE ARNETT
LEAD RESEARCH SCIENTIST
Ex-UC San Diego
Multiple papers accepted at EMNLP
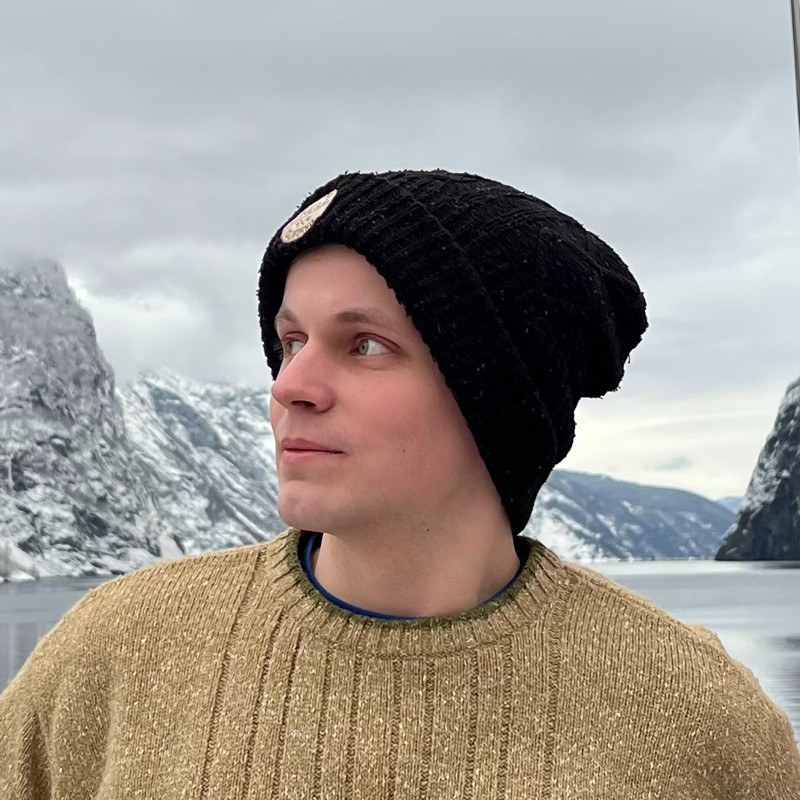
PAVEL CHIZHOV
LLM ENGINEER
University of Würzburg/University of Tartu, CS/Vision/NLP
Ex-Yandex
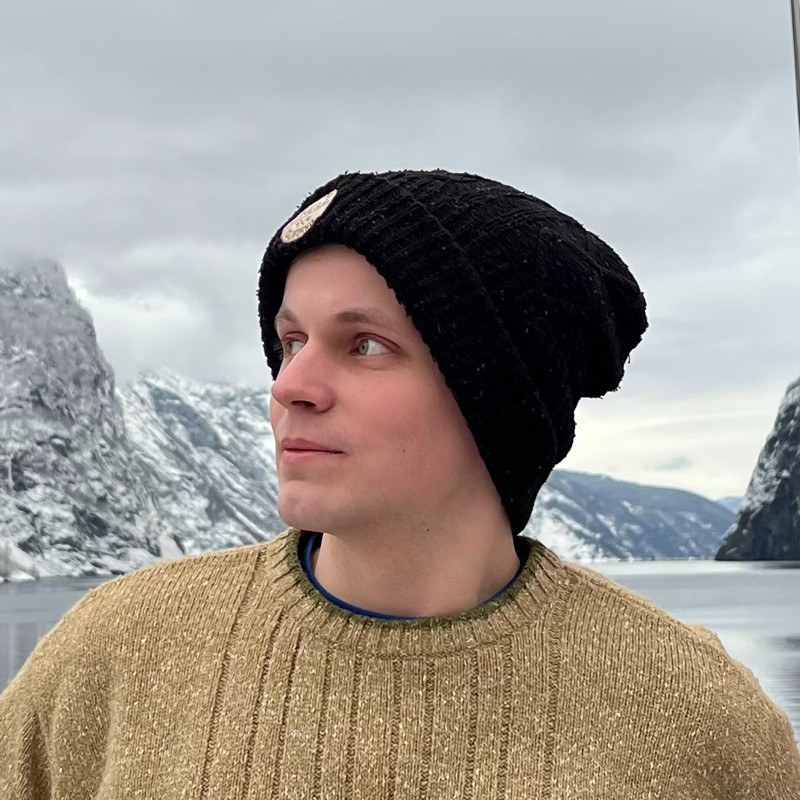
PAVEL CHIZHOV
LLM ENGINEER
University of Würzburg/University of Tartu, CS/Vision/NLP
Ex-Yandex
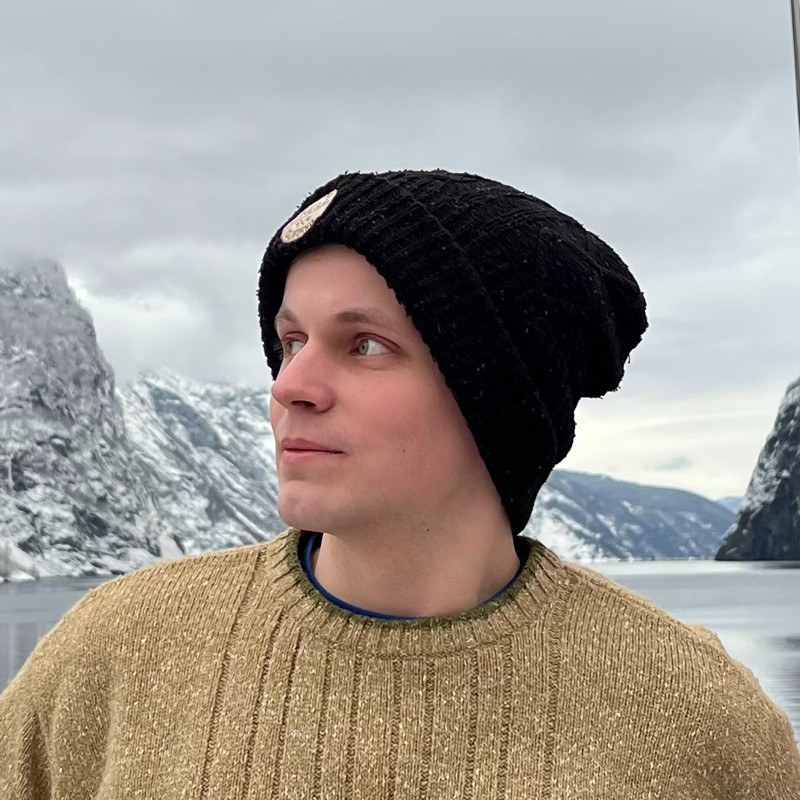
PAVEL CHIZHOV
LLM ENGINEER
University of Würzburg/University of Tartu, CS/Vision/NLP
Ex-Yandex
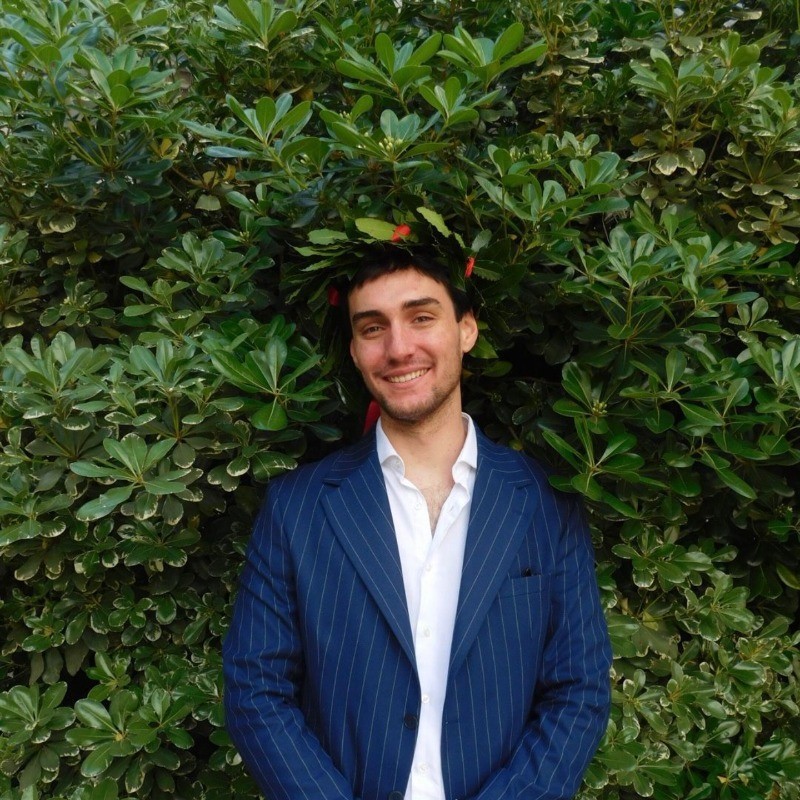
MATTIA NEE
LLM SCIENTIST
Ex-University of Milan
Fundamental Mathematics/NLP
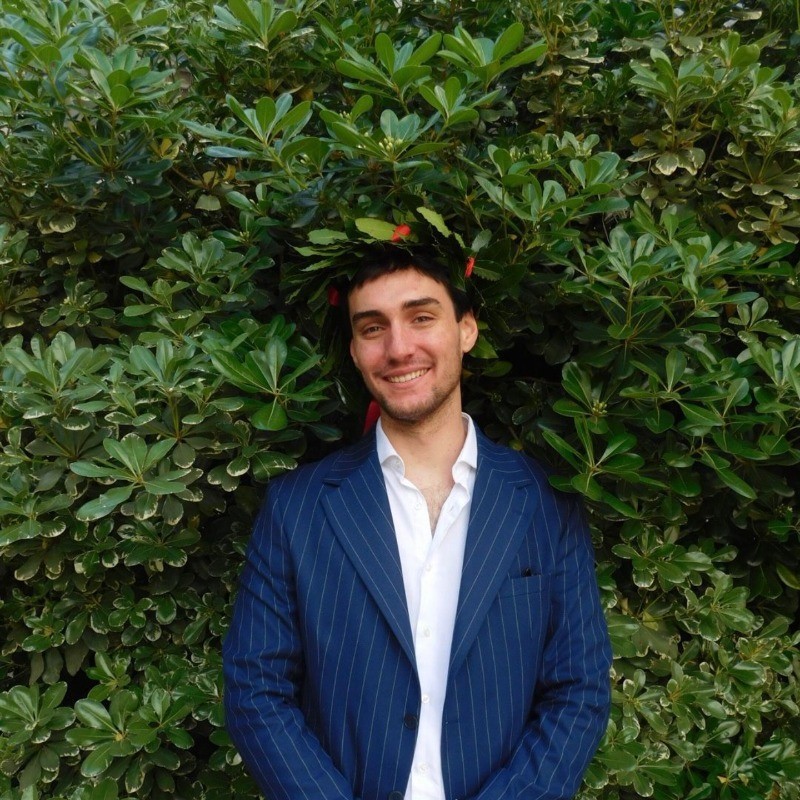
MATTIA NEE
LLM SCIENTIST
Ex-University of Milan
Fundamental Mathematics/NLP
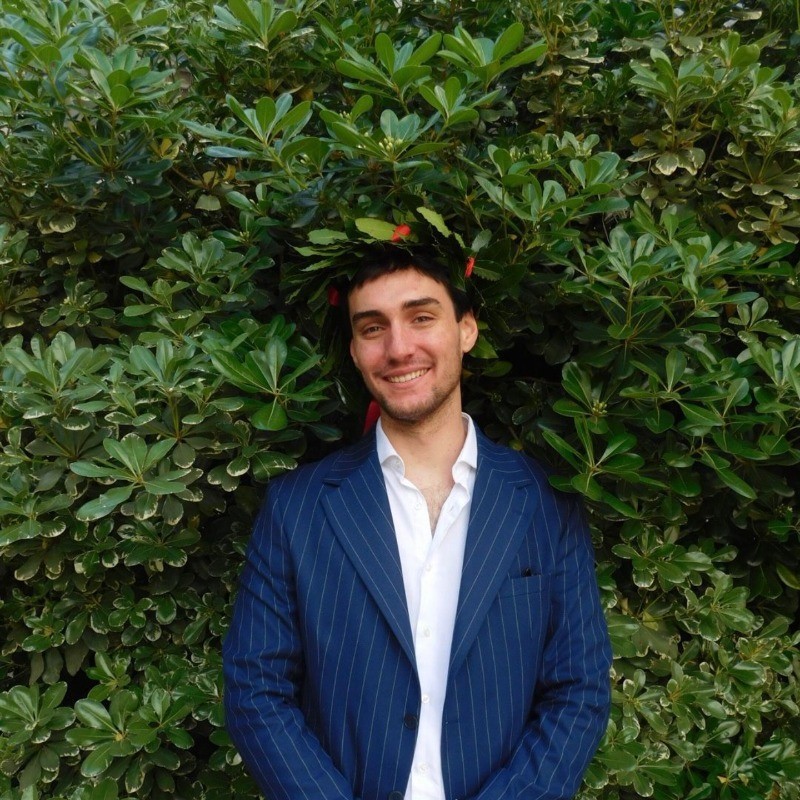
MATTIA NEE
LLM SCIENTIST
Ex-University of Milan
Fundamental Mathematics/NLP
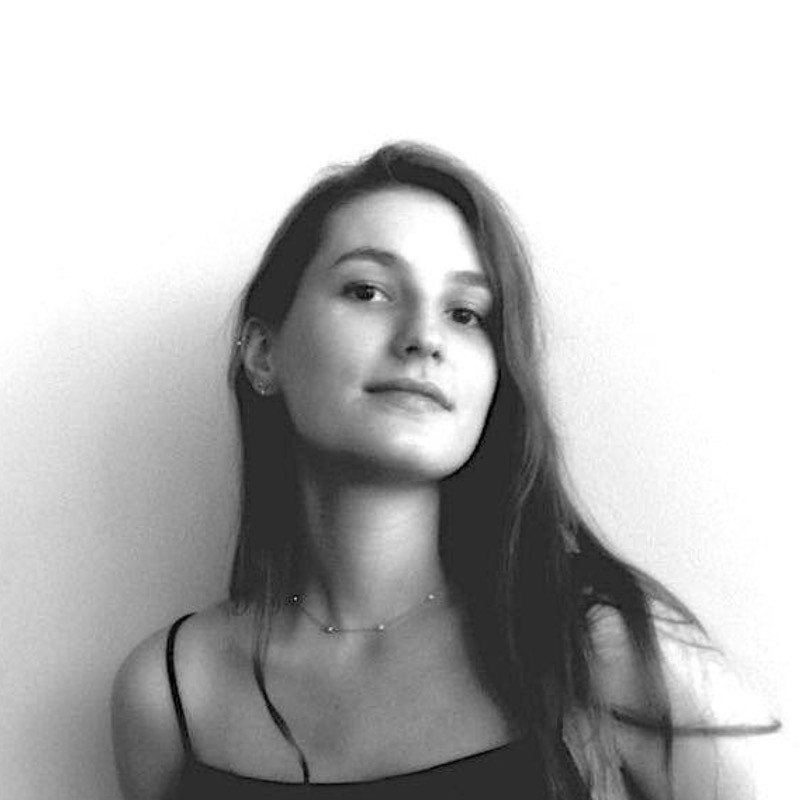
EKATERINA KOZACHENKO
LLM SCIENTIST
University of Lorraine
ETH Zürich, NLP
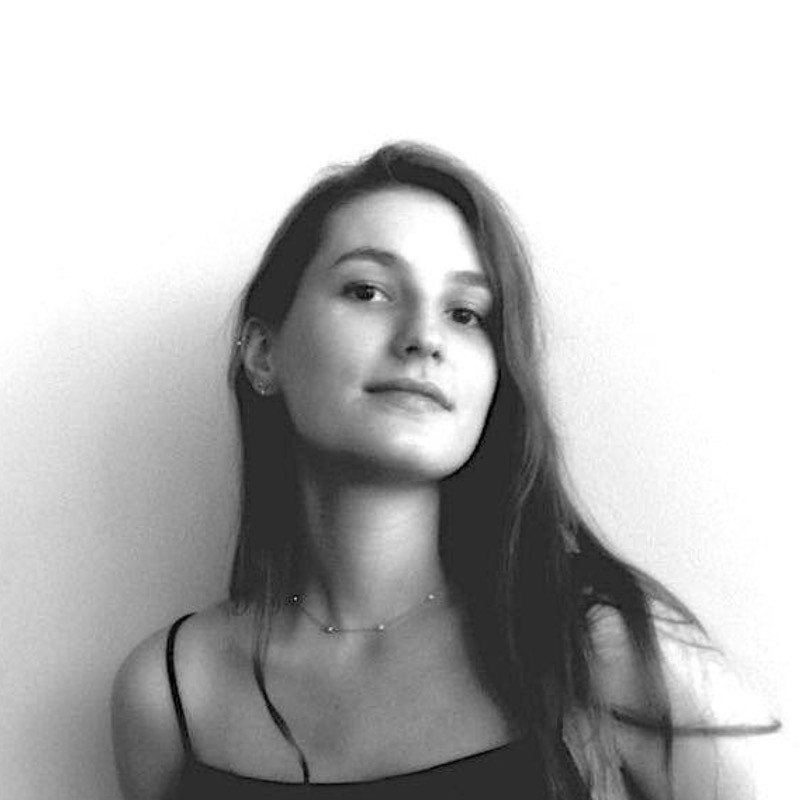
EKATERINA KOZACHENKO
LLM SCIENTIST
University of Lorraine
ETH Zürich, NLP
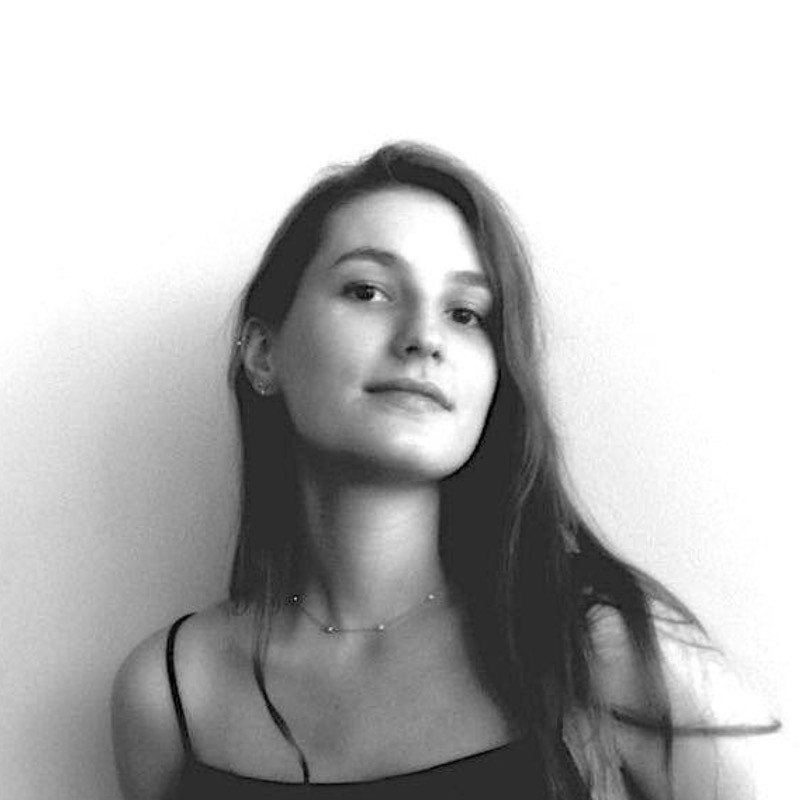
EKATERINA KOZACHENKO
LLM SCIENTIST
University of Lorraine
ETH Zürich, NLP
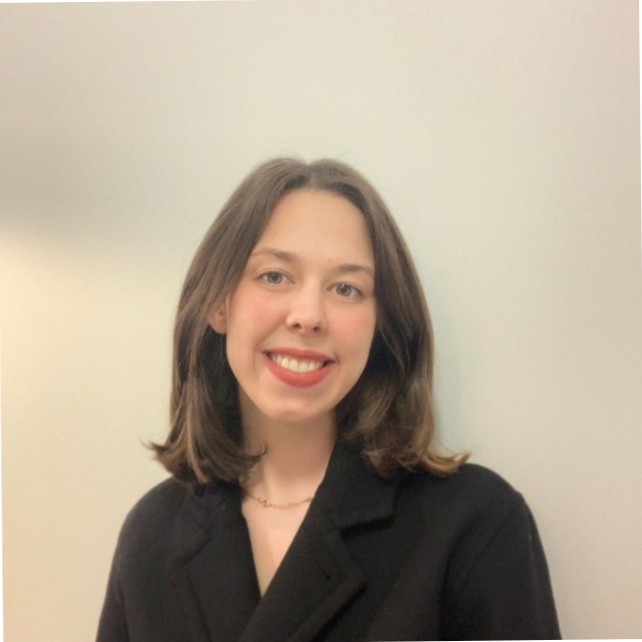
IRÈNE GIRARD
DATA ANALYST
Ex-Sciences Po
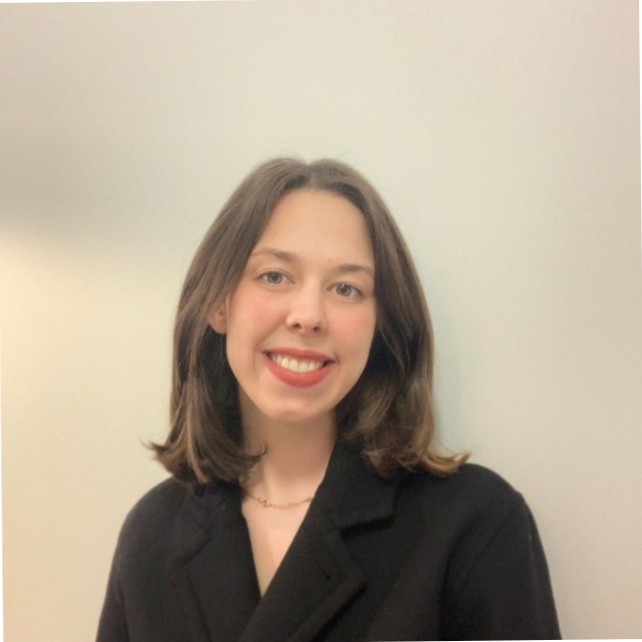
IRÈNE GIRARD
DATA ANALYST
Ex-Sciences Po
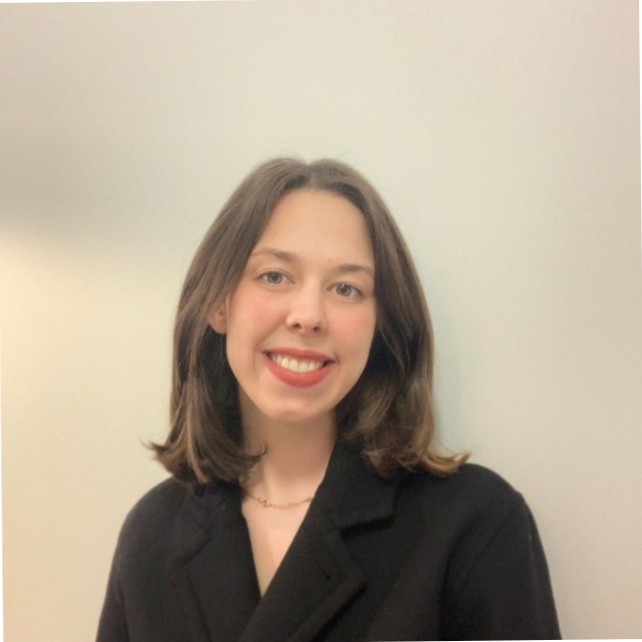
IRÈNE GIRARD
DATA ANALYST
Ex-Sciences Po
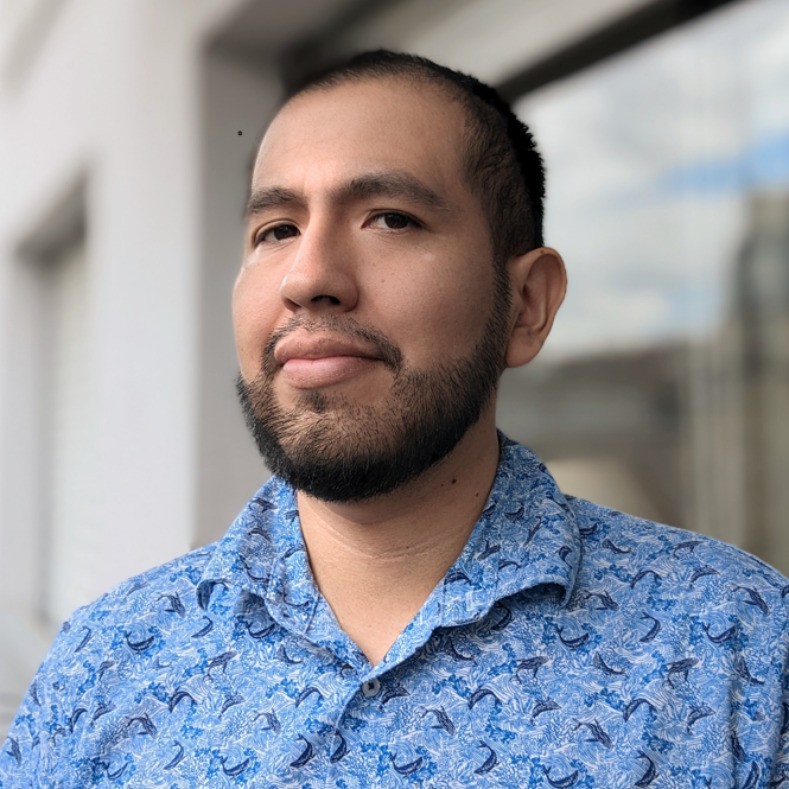
CARLOS ROSAS
SENIOR DATA SCIENTIST
Ex-Sorbonne Université
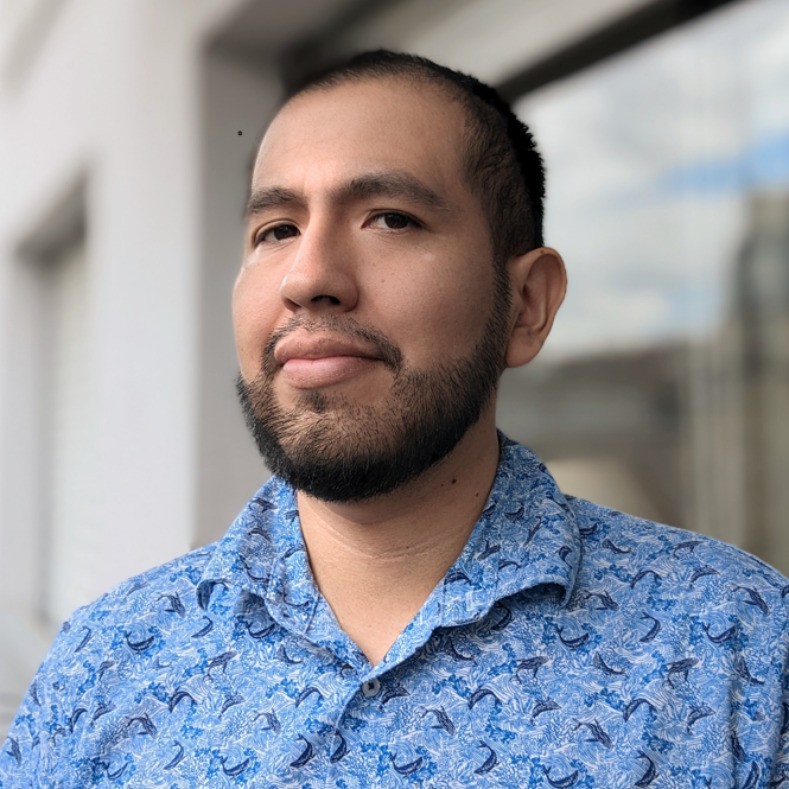
CARLOS ROSAS
SENIOR DATA SCIENTIST
Ex-Sorbonne Université
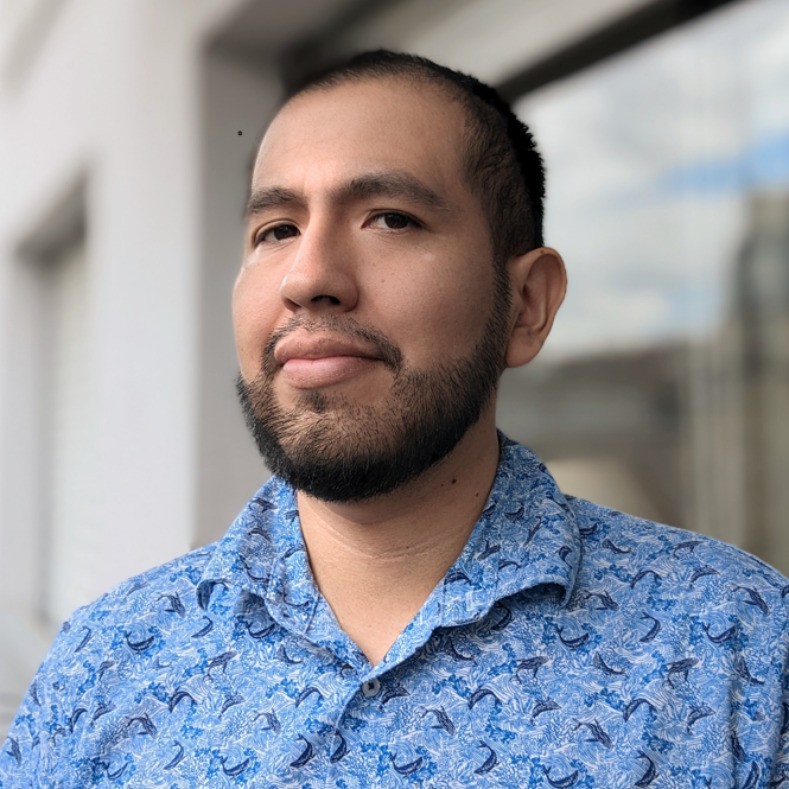
CARLOS ROSAS
SENIOR DATA SCIENTIST
Ex-Sorbonne Université
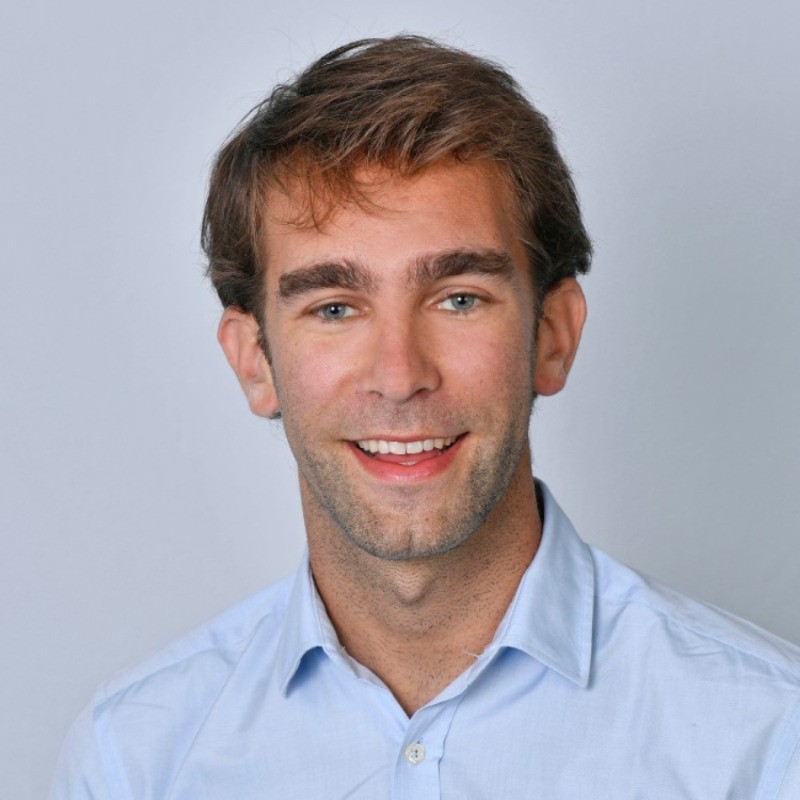
MATTHIEU DELSART
STRATEGY & IMPLEMENTATION LEAD
HEC / Polytechnique
Ex-Alan Ex-Artefact
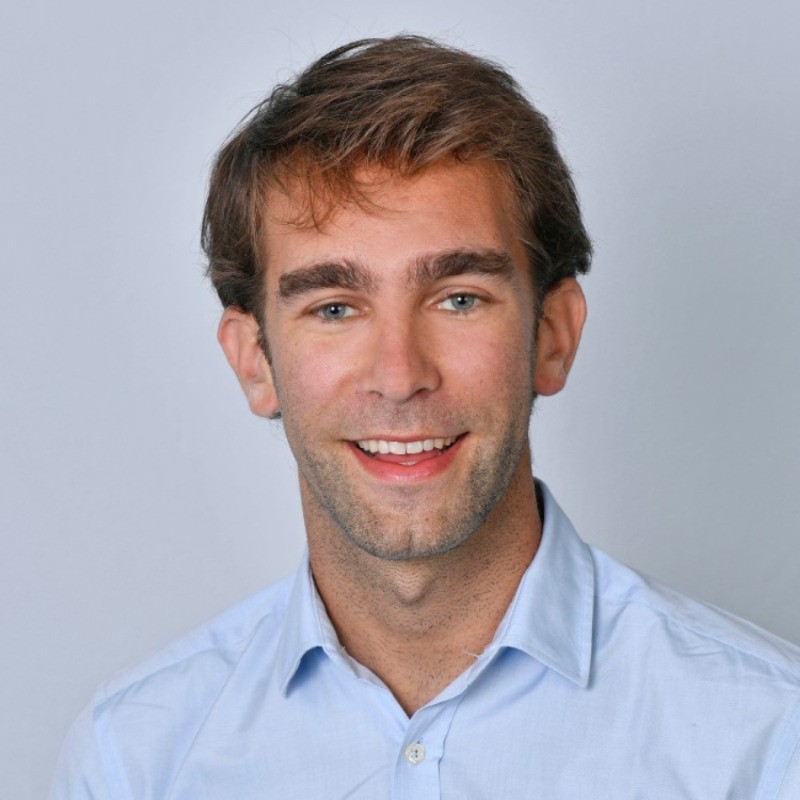
MATTHIEU DELSART
STRATEGY & IMPLEMENTATION LEAD
HEC / Polytechnique
Ex-Alan Ex-Artefact
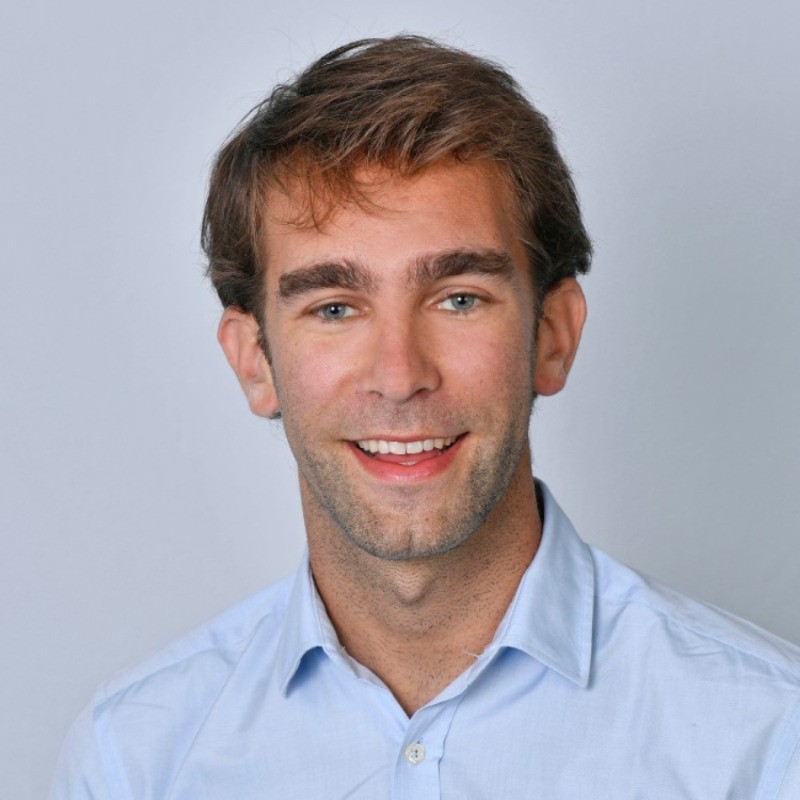
MATTHIEU DELSART
STRATEGY & IMPLEMENTATION LEAD
HEC / Polytechnique
Ex-Alan Ex-Artefact
OUR PARTNERS
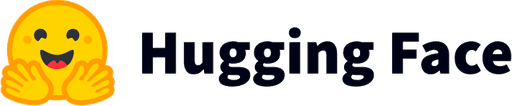
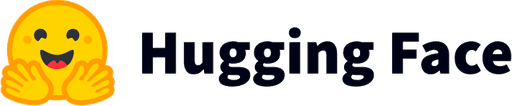






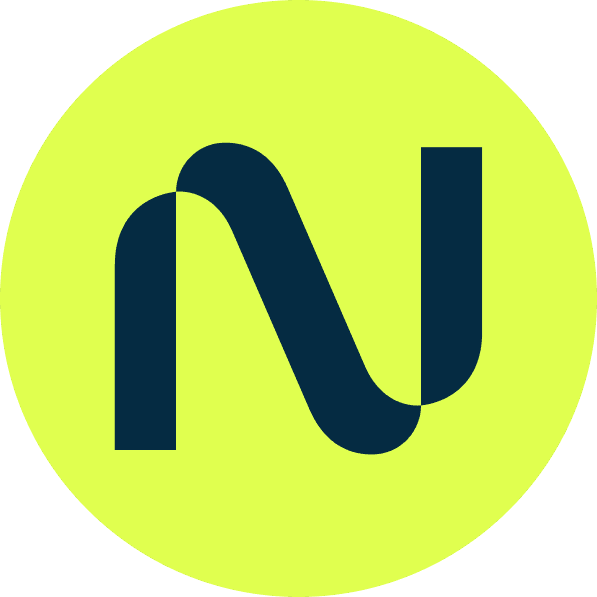
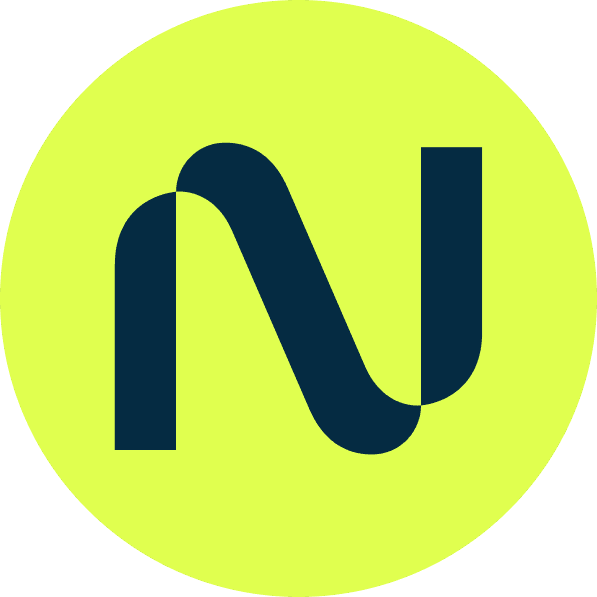
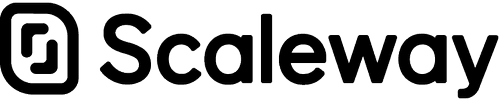
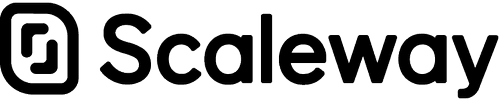
Member of Scaleway Startup Growth Program


Member of the Inception Program
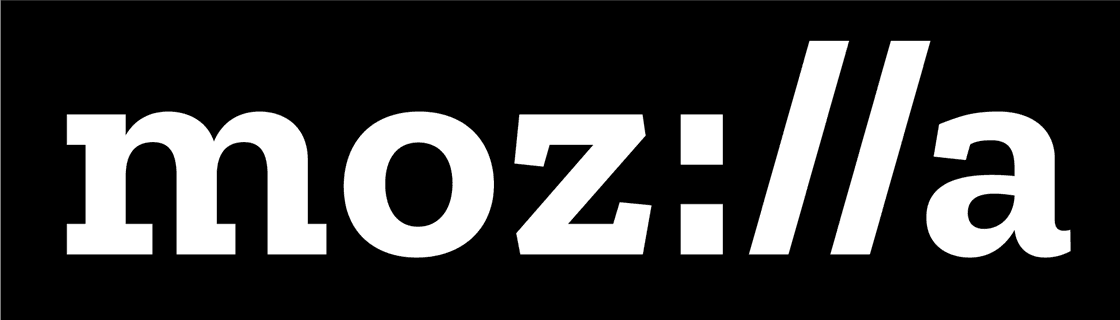
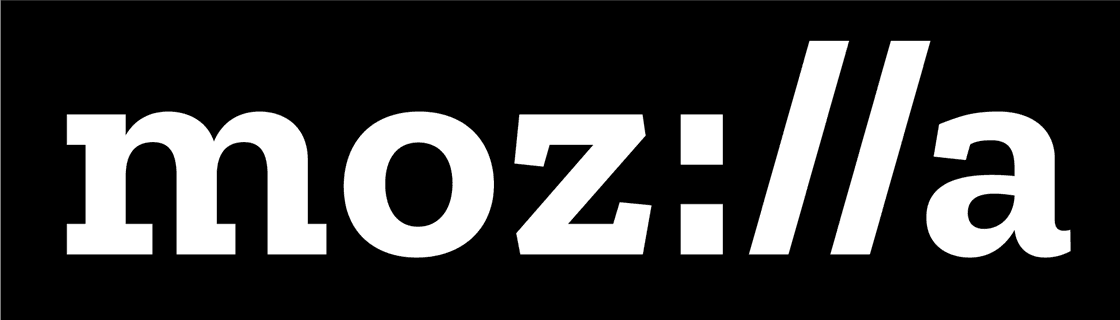
Local AI Builders
RESEARCH
BPE Gets Picky: Efficient Vocabulary Refinement During Tokenizer Training
Pavel Chizhov, Catherine Arnett, Elizaveta Korotkova, Ivan P. Yamshchikov
Language models can largely benefit from efficient tokenization. However, they still mostly utilize the classical BPE algorithm, a simple and reliable method. This has been shown to cause such issues as under-trained tokens and sub-optimal compression that may affect the downstream performance. We introduce Picky BPE, a modified BPE algorithm that carries out vocabulary refinement during tokenizer training. Our method improves vocabulary efficiency, eliminates under-trained tokens, and does not compromise text compression. Our experiments show that our method does not reduce the downstream performance, and in several cases improves it.
Language models can largely benefit from efficient tokenization. However, they still mostly utilize the classical BPE algorithm, a simple and reliable method. This has been shown to cause such issues as under-trained tokens and sub-optimal compression that may affect the downstream performance. We introduce Picky BPE, a modified BPE algorithm that carries out vocabulary refinement during tokenizer training. Our method improves vocabulary efficiency, eliminates under-trained tokens, and does not compromise text compression. Our experiments show that our method does not reduce the downstream performance, and in several cases improves it.
Toxicity of the Commons: Curating Open-Source Pre-Training Data
Catherine Arnett, Eliot Jones, Ivan P. Yamshchikov, Pierre-Carl Langlais
Open-source large language models are becoming increasingly available and popular among researchers and practitioners. While significant progress has been made on open-weight models, open training data is a practice yet to be adopted by the leading open-weight models creators. At the same time, there researchers are working to make language models safer. We propose a data curation pipeline to reduce harmful outputs by models trained on public domain data. There are unique challenges to working with public domain data, as these sources differ from web text in both form and content. Many sources are historical documents and are the result of Optical Character Recognition (OCR). Consequently, current state-of-the-art approaches to toxicity filtering are often infeasible or inappropriate for open data models. In this paper, we introduce a new fully open-source pipeline for open-data toxicity filtering. Our contributions are threefold. We create a custom training dataset, ToxicCommons, which is composed of texts which have been classified across five different dimensions (racial/origin-based, gender/sex-based, religious, ability-based discrimination, and violence). We use this dataset to train a custom classifier, Celadon, that can be used to detect toxic content in open data more efficiently at a larger scale. Finally, we describe the balanced approach to content filtration that optimizes safety filtering with respect to the filtered data available for training.
Open-source large language models are becoming increasingly available and popular among researchers and practitioners. While significant progress has been made on open-weight models, open training data is a practice yet to be adopted by the leading open-weight models creators. At the same time, there researchers are working to make language models safer. We propose a data curation pipeline to reduce harmful outputs by models trained on public domain data. There are unique challenges to working with public domain data, as these sources differ from web text in both form and content. Many sources are historical documents and are the result of Optical Character Recognition (OCR).
Consequently, current state-of-the-art approaches to toxicity filtering are often infeasible or inappropriate for open data models. In this paper, we introduce a new fully open-source pipeline for open-data toxicity filtering. Our contributions are threefold. We create a custom training dataset, ToxicCommons, which is composed of texts which have been classified across five different dimensions (racial/origin-based, gender/sex-based, religious, ability-based discrimination, and violence).
We use this dataset to train a custom classifier, Celadon, that can be used to detect toxic content in open data more efficiently at a larger scale. Finally, we describe the balanced approach to content filtration that optimizes safety filtering with respect to the filtered data available for training.
PRESS
CONTACT@PLEIAS.FR
CONTACT@PLEIAS.FR
CONTACT US
We build energy-efficient LLMs for information-intensive and highly-regulated industries.
© 2024 PleIAs
Website by Themost Studio©
UP