Train Green, Train Strong
Jan 21, 2025
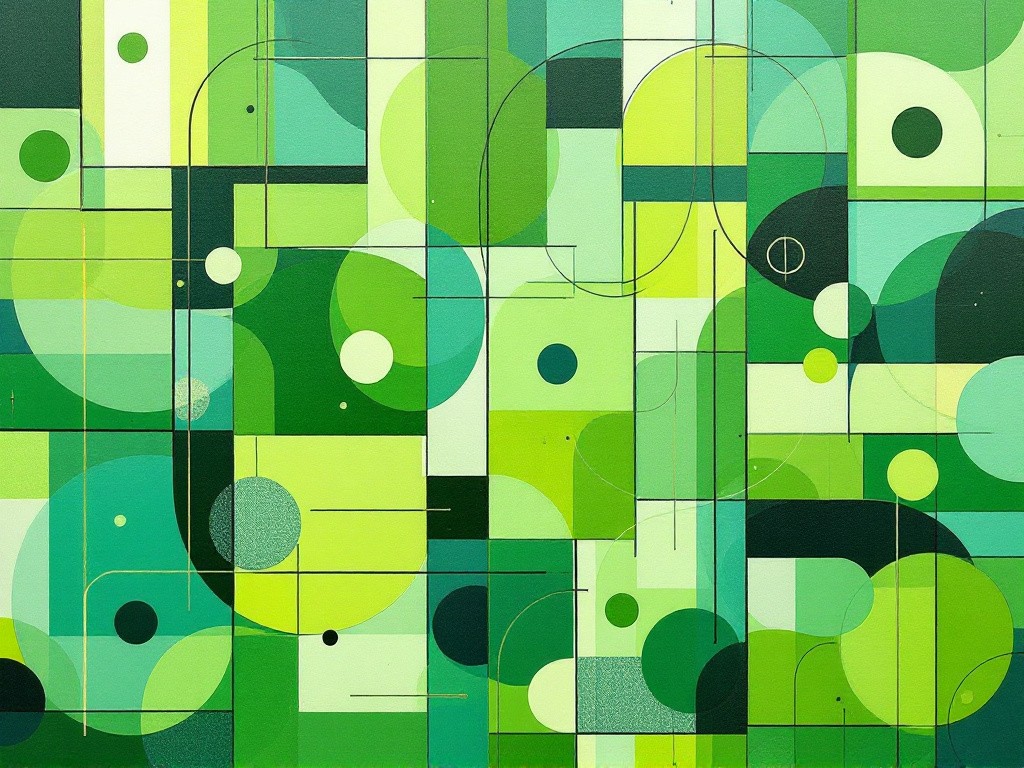
Ecological Impact of Inference Scaling
The release of O3 raises many questions about the increased energy and ultimately ecological impact of this new approach of "inference scaling". OpenAI seems to have circumvented the data wall by letting the model think for much longer, writing multiple if not thousands of drafts before settling on an answer.
In reality we don’t know. OpenAI provides the cost for the low compute tasks ($20 each). We only know the high compute tasks are 172 times more compute intensive as it involves the creation of 1024 drafts instead of 6. What is still undetermined:
How effective is OpenAI grid compute? They are unlikely to use your standard H100 install.
Do compute costs raise linearly? There could be many opportunities for parallelization.
What is the energy mix? Fossil fuels, nuclear?
The total amount of CO2 per high compute task can at best be approximated. Boris Gamazaychikov made one of the best conservative estimates so far: 3600 per high compute tasks (maybe less depending on the level of optimization) which would represent around 1700-1800 kWh.
Lack of Transparency
The lack of transparency in the AI industry makes it hard to have a well-informed debate. Computers and grid infrastructures have always been good at optimizing. Many frequent recommendations to reduce consumption are not effective at all (you can stop deleting your mails). We have seen a similar patterns for AI inference cost, as models have reduced dramatically in size over the past two years. Obviously this may not be enough to offset the rebound effect, as due to lowering costs, uses becomes much more widespread.
I’m way more preoccupied right now by the market trends, and that goes beyond OpenAI. Investors are overtly betting on the emergence of high end AI product for corporations. There will be a wave of new "agentic" applications with enough capital to tap into pricey subscriptions from model providers. Inference scaling can support a new economy of "deluxe" ChatGPT with much increased consumption costs that will not necessarily discourage end users, either due to genuine automation gains in some fields like SWE or speculation.
Small Language Models: a Green Alternative
This is not a fatality. At Pleias we are training small and frugal language models for specialized use case like RAG. Specialization and model design for infrastructure integration brings many opportunities for reduced consumption. We have been surprised recently to see how models perform better on some metrics than GPT-4, like citation accuracy/hallucination.
If generative AI is on course to become a more mature technology, it may be time to collectively use our thought tokens and realize cost is not all about money.